Design of experiments: an introduction
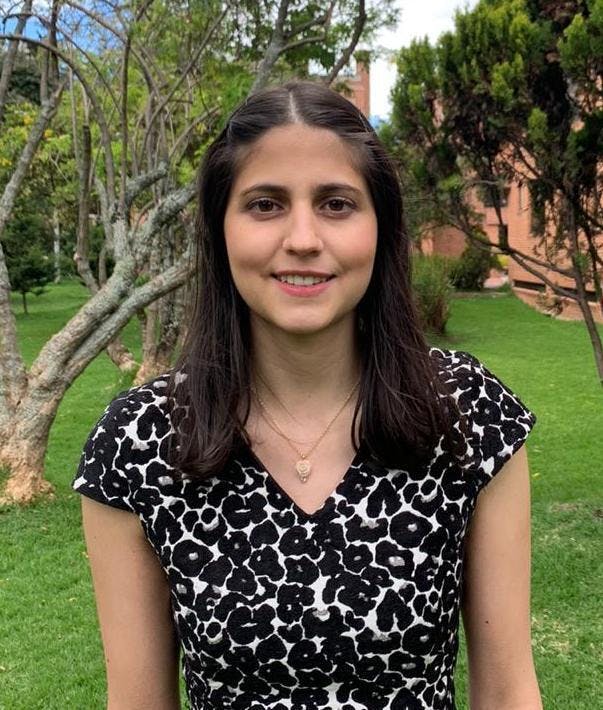
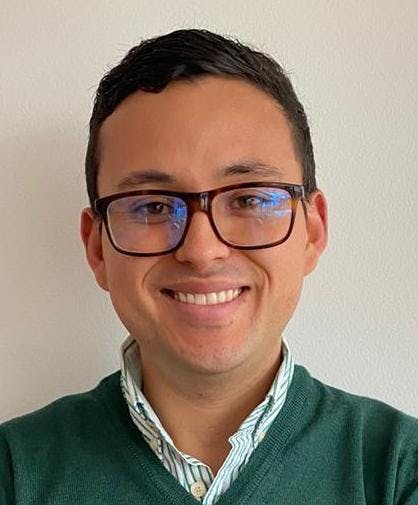
Paula Pico
Juan Pablo Valdes
Overview
This blog post showcases the benefits of a design-forward mindset in various industries. We summarise the basics of Design of Experiments (DoE) and provide starting-point examples for non-experts, while also illustrating through short success stories the real-life advantages of integrating and adopting DoE techniques at any level in your organisation. We also highlight the benefits of using the Quaisr platform for creating DoE workflows.
Increasing quality through design-thinking — DoE in your organisation
The concept of ‘design’ is embedded in everything scientists and engineers do on a day-to-day basis, from Research and development (R&D) undertakings to quality control, process optimisation, physics- and data-driven modelling, prototyping, and statistical analysis. DoE is a set of statistics-based methodologies aimed at evaluating the individual or interactive factors that influence a system’s outcome. Identifying this cause-and-effect relationship through random experimentation can be a daunting and costly task, not to mention potentially unreliable and markedly prone to all sorts of biases. Alternatively, DoE provides a systematic framework to plan your experimentation in a way that maximises insights from each experiment. DoE also focuses on the validity, replicability, and reproducibility of the obtained data outputs. DoE-based methods have been successfully applied in a multitude of industries, such as drug formulation and manufacturing (more on this later), food flavour and sensory analysis for consumer goods, chromatography, and the design of energy-efficient buildings.
A few general examples of what DoE can do in your organisation are the following:
-
Aid in the selection/evaluation between alternatives (i.e. comparative experiments): DoE methods can identify the most appropriate set of experiments to solve the problem of choosing between competing alternatives. DoE can answer questions like: which synthesis protocol would result in a higher product yield? Does this new additive improve the reaction outcomes over the current one? Which of our main products should we prioritise? Which impeller geometry should we select for our industrial mixer? A successful comparative experiment will have a well-defined set of performance metrics by which each alternative will be assessed via a sampling of its response to the conditions set by the experiments.
-
Identify which factors/effects govern a given process/system (i.e., screening design methods): these methods are useful when our system of interest is surrounded by a large pool of factors that may or may not have an influence over its response. Because the dominating factor in our system is not readily identifiable, controlling our system’s response becomes a difficult task. Screening design helps by sorting out the statistically significant factors from the ones that have little to no effect. Considering the complex phenomena governing the dynamics of an industrial-scale production plant, one could think of a large list of potential factors (operating temperature of each unit, inlet-outlet flow rates, reactor residence times, properties of the raw materials, equipment setpoints, maintenance practices, among others) that could influence, say, the purity of your main product. DoE techniques sift through all these parameters and identify the ones that are best correlated with the system’s outcomes of interest.
-
Search for a maximum/minimum or specific process output to improve robustness and reduce variability in the operation (i.e., response surface and regression modelling): once the primary factors responsible for the system’s behaviour have been identified, response surface modelling guides the sampling over the multi-parameter space in a way that will allow us to estimate how inter-parameter interactions influence the system’s outcomes. As such, our sampling will produce data that can be fitted to a response surface model and employed to locate points of optimal operation.
The real-life impact of DoE
Now that we’ve got the basics down, let's dive into a few real-life scenarios where DoE has generated quantifiable industrial impact.
Quality-by-design (QbD) in pharmaceuticals: getting it right from the get-go
The pharmaceutical industry nowadays bases its design operations on the principle of ‘quality by design’. This means that the quality of the final product is targeted and built into the product from the design and manufacturing stages instead of being assessed after production. For QbD to be successful in a given organisation, a deep understanding of the physico-chemical mechanisms governing the process ought to be coupled with high-quality risk management and statistically-sound experimentation practices, such as DoE. QbD came at a time when nearly all problems related to poor quality in the pharmaceutical industry were directly tied to the design stages1 ; the consequences of these problems can amount to costs reaching 40% of the company's total revenue.2 Since its wide adoption, QbD has allowed pharmaceutical companies to exert higher control over their operations by increasing consistency and reliability in drug production and reducing operational downtime.
PET Scanners in the fight against cancer and other diseases
Positron emission tomography (PET) scans are imaging tests that provide a 3-D visualisation and measurement of the biochemical activity of tissues and organs in the human body. Their results are used for detecting abnormal cellular activity, allowing them to identify signs of various types of cancer, brain disorders, and heart problems, including heart attacks and coronary artery disease3. Central to the operation of PET scans is the injection of a radioactive tracer into the patient’s bloodstream that binds to a specific part of the body, such as a tumor. The scanner’s scintillator crystal-based detection system can detect the photons emitted from a positron emission and annihilation process3 and trace their source back to the body. Given the paramount importance of this technology, the field is in continuous search for improvements to the frequency of detection and image quality, as well as reducing the tracer doses to unlock its viability for a broader range of patients, including young children. Achieving these goals, however, does not come easy as the optimal design of the detection crystals is immersed in an interacting multi-dimensional space that involves various molecular-scale crystal properties. Because of this, one-at-a-time experimental and simulation runs are prohibitively numerous and costly. This is where DoE, together with uncertainty quantification techniques and surrogate modelling has led to 20 times faster scanning times and higher image sensitivity. 5
Understanding your consumers: DoE in Fast-moving Consumer Goods (FMCG)
The FMCG industry faces a unique series of challenges given the fast-paced and competitive nature of its market. In it, the product design needs to be constantly updated to keep up with the ever-evolving consumer preferences and behavioural shifts. With the dramatic changes brought about by the COVID-19 pandemic, consumers demand more personalised products, tailored buying experiences6, and sustainable production, requiring enormous innovation, process optimisation, and consumer studies efforts. It is estimated that non-durable goods companies, including FMCG, account for around 23% of the total worldwide expenditure in market and consumer research, which, in 2015, amounted to about $16 billion. 7 Companies in the field are rapidly moving towards the adoption of a more systematic and scientific approach to carry out their market and consumer research. Guided by experimental design, marketing teams in FMCG companies are planning and executing behavioural studies on their consumers, motivated by the long history of successful deployment of DoE in R&D. This allows them to understand the consumers’ response to marketing stimuli and to gather information about their conscious or subconscious preferences. This incredibly valuable information is being used as a driver for innovation in the consumer goods R&D. A further look into DoE-based consumer studies can be found here.
How do we actually design our experiments? — Common DoE methods
Before jumping blindly into any given DoE, we must first consider: What are the experiments’ objectives? Certainly non-trivial, it is crucial to identify and prioritise objectives to establish and manipulate the right set of input and output parameters. These variables follow a specific terminology within DoE practices:
Factors: are the set of input parameters that have an effect on the measured output variables. They can be subdivided in two categories, and treated accordingly in the chosen design framework (e.g., blocking and randomisation).
- Controllable: input parameters that can be modified or controlled at will in the experiment/process (i.e., quantity of rice and water in a rice cooking process).
- Uncontrollable: parameters (e.g., external source or noise) that cannot be changed or controlled, but still affect the measured outputs (i.e., atmospheric pressure when cooking rice).
Responses: Measured (output) process variables that gauge the desired effect or outcome.
Factors can be identified and structured hierarchically via cause & effect diagrams (e.g., Fishbone diagrams). Each factor comes with a set of descriptors known as ‘levels’, which specify the range of values adopted during the experimental runs. Critical engineering judgement is required to avoid infeasible or impossible scenarios when setting up different test cases (i.e., evaluating temperatures above the boiling point or below the freezing point in a system that is meant to be in liquid state). Additional DoE concepts worth mentioning are as follow:
Interaction: refers to a scenario where the effect of one factor on a response depends on the level of one or multiple other factors (n-level interaction).
Treatment specific set of factor levels to be compared against another treatment.
Confounding: indicates that the effect of a factor on a response may come from the factor itself and also from contamination or bias from higher order interactions.
Resolution: describes the degree to which the effect of a single factor is confounded with n-level interactions.
Once the dust has settled, we can jump into a DoE framework to determine the arrangement of experiments that can be tailored to our defined objectives. Let's explore the theory and implementation of some of the most common DoEs using Python.
Factorial designs:
Probably the most common starting point when looking at a system that holds two or more factors are factorial designs, which can be broken down into two subcategories:
- Full design, where the set of experiments will take on all possible combinations,
- Fractional design, where a subset of the full design is carefully chosen.
The full factorial design allows us to examine the effect of the main factors, as well as the effect of the interactions between factors. For instance, we can explore the effect of oven temperature, amount of flour and baking time on the consistency of a cake, as well as the difference between raising the oven’s temperature for shorter or longer baking times, or finding the temperature at which the amount of flour is not relevant (your cake might be overcooked at this point!).
Commonly, factorial designs consider 2-level factors (known as 2k, where k stands for number of factors), but can be implemented for more complex cases with 3 or more levels (see here for further information on 3k designs). Lets see how we can obtain a 2k design for the cake baking example through the DOEPY python library:
A similar output can be obtained from other DOE libraries such as pyDOE2 by running the following commands:
Although a bit more jargony, pyDOE2 may be advantageous when carrying out further computations since the factors (given as columns) are already coded in standard notation, with +1 and -1 for high and low levels respectively.
When handling a larger number of factors, research may become logistically infeasible. For these cases, it is best to perform a fractional factorial design, commonly notated as 2(k-n), where k stands for the number of factors and n describes the size of the fraction of the full design. An important downside is the concept of confounding, where factors' main effects get entangled or ‘muddled’ with interaction effects, making it harder to distinguish between them (further info on this topic can be found here).
Let’s suppose we cannot run all 8 experiments proposed above, so we propose a 2(3-1) (half factorial) with only 4 runs. By setting the alias “C=AB”, we are defining factor C to be confounded with the interaction effect of factors A and B. By running the fractional design builder from pyDOE2, we get the following output:
We can readily verify from the output that the levels for factor C (third column) have been calculated from the product of the corresponding levels for A and B. Let’s now consider three extra factors in our cake baking scenario: sugar mass, cooling time and butter mass. Another way of building a fractional design is by setting its resolution, which refers to the ability to separate main effects from low order interactions. As one might expect, low resolution designs are not particularly useful since main effects get confounded between each other. Our baking scenario with a resolution of 3 generates the following output:
In such a case, we are able to identify main effects but there might be confounding two factor interactions. If we increase the resolution we remove this constraint, but consequently we jump from 8 to 16 experiments. Plackett-Burman designs can be an alternative way of approaching fractional designs when handling numerous factors (6+).
Randomised designs - Latin hypercube sampling:
Randomised designs are fitted to work with one main factor of interest while isolating other factors which affect the response but are not necessarily of interest at first (e.g., ‘nuisance’ or noise variables). Latin hypercube sampling (LHS) in particular is a statistical method for generating a quasi-random sample of parameter values from a multidimensional distribution, where each sample is unique in each axis-aligned hyperplane containing it (generalisation of Latin squares to an arbitrary number of dimensions). LHS grants a more uniform spread of random points from the sample space by first subdividing it in smaller cells and choosing only one element per cell. Going back to our cake baking example, let’s construct a simple and space-filling Latin hypercube through the DOEPY library, specifying a density of 12 sample points:
Although at first glance both methods seem to construct a similarly random design, space-filling LHS attempts to create sample points everywhere in the experimental region with as few gaps as possible. This is achieved through different optimisation approaches (i.e., maximising the minimum distance among points), which avoids the generation of poor LH designs (i.e., sampling all points over the same diagonal).
pyDOE2 performs a similar operation (coded with 0 to 1 values for each factor’s level) but allows us to specify how sample points will be selected (e.g., centre points, max. distance between points, etc).
Response surface - Central composite design:
Response surface designs are especially useful when attempting to build a second order model for the response variable (estimating interaction and quadratic effects) without the need to implement a full three-level factorial design. The Box-Wilson or central composite design consists of a set of three experimental units: an embedded 2-level (fractional) factorial design, a set of centre points (median values of the factorial runs) and a set of axial points (same centre points but taking extreme values for one factor). This can be pictured as a centre-point factorial design augmented with ‘star’ points, which allows the design to capture quadratic behaviour. As for previous designs, the central composite requires a dictionary specifying the factors and levels, as well as the specific type of design (i.e., circumscribed ‘ccc’, inscribed ‘cci’ or faced ‘ccf’, for more information refer to this article).
For DOEPY:
And similarly for pyDOE2:
Quaisr links DoE throughout your digital environment
Organisations need to implement DoE practices to improve their design process efficiency, reliability, and sustainability. However, many are not prepared to update their digital environment and physical processes with design-thinking practices. Recent studies have pointed out that over 70% of business leaders and decision-makers believe their organisation doesn’t have a clear path towards digitalisation. What's more, 85% in the UK (out of 600 surveyed) recognised the importance of digital advancement now more than ever before 8.
Quaisr creates, connects and consolidates your digital environment, allowing organisations to seamlessly embed DoE methods throughout your processes, developing your workflow around design-thinking practices. No need for a steep and costly learning curve with extra steps. Quaisr navigates IT complexity without upskilling in deployment, so engineers can focus on being engineers!
Quaisr links DoE with physics modelling
Quaisr is a model/tool agnostic platform, which means there are very few deployment restrictions for library type, model or simulation package. It is as simple as creating and connecting blocks within an intuitive, user-friendly, and easy to visualise drag-and-drop environment. Many commercial simulation packages nowadays include DoE methods to some extent, but their flexibility and availability might not be sufficient to tailor to your specific needs. Such would be the case of an experiment involving multiple simulations connected in series, where the DoE needs to be done on the overall workflow, rather than just acting as an isolated block in the workflow. What’s more, multidisciplinary teams will struggle to scale a single modelling package amongst entirely different processes, and the steps needed to integrate DoE libraries with any given modelling software, whether commercial, open-source or in-house, is certainly not a straightforward task.
All of this brings forth numerous pressing issues:
- Implementing and managing different modelling packages simultaneously can be a cumbersome and sometimes nearly-impossible task.
- Responding swiftly to today’s dynamic market will be extremely difficult with a non-adaptive and poorly-connected simulation/process modelling framework.
With Quaisr, blocks can be fully customised, allowing you to make the best out of both worlds: interconnecting familiar/proven commercial packages with process-specific open-source or in-house libraries. Quaisr automates simulation deployment by adhering to design-thinking practices, allowing engineers and scientists to plan efficient and cost-effective simulation runs, a crucial aspect in today’s world where the demand for computational resources keeps escalating tremendously.
Quaisr links ingenuity, bringing experts and non-experts together
A major hurdle engineers and scientists face is the extensive range of knowledge and skills demanded by today’s highly intricate and ever-evolving processes. This reality places major strain on organisations, spreading their experts too thin, leading to inefficient process development, and falling behind in the present-day competitive market. A recent survey conducted by Microsoft on employees and business decision-makers has found that over 70% of them find it hard to share information across their company, with 77% stating that data is siloed across teams and departments. 9
Quaisr’s inherent connectivity empowers teams throughout your organisation to collaborate and effortlessly share knowledge. Through the platform, expert teams in DoE and statistical analysis can link up with marketing and consumer outreach teams to best design a joint plan. There is no need for engineers to learn the “language” of marketing or vice versa. Similarly, experimental and modelling research teams can link up and work in a coordinated fashion, transferring the necessary skills to contribute effectively to the design plan. Quaisr provides the autonomy engineers need to do what they do best: be engineers.
Quaisr lets you collaborate and keep accountability
The benefits of an interconnected mode of operation are surely appealing to virtually any type of organisation. Nonetheless, new challenges to the organisation are guaranteed to surface as additional layers of complexity are added to its digital environment. For example, as the data-powered predictions of the data analytics team are linked to the first-principle models of the engineering and research department, the number of parameters and available models capable of altering the outcome of a certain set of simulations increases substantially. If we connect this to the areas in charge of applying design-thinking through DoE techniques, it is easy to see how the entire framework could spiral out of control without a reliable and robust tracking of all aspects comprising the digital environment. This because of the involvement of multiple people using a multitude of different softwares and models. Quaisr’s platform keeps your organisation away from these issues by providing an easy way to centralise and monitor the inputs and outputs generated by various teams. This includes a built-in version control of the simulations, a detailed history log of all changes made, as well as the possibility of reverting back to previous simulation iterations, increasing auditability, governance, and accountability.
Quaisr lets you reliably add complexity over time
Quaisr takes connectivity even further. On top of simulation automation, DoE methods can help engineers build training datasets for data-driven surrogate models. Let’s consider a physics-informed data-driven model that seeks to optimise an industrial mixing operation from its geometry and operational constraints. Normally, such a model would be tuned with high-fidelity Computational Fluid Dynamics (CFD) simulations, which can be extremely costly and lengthy to run at the level of detail required, taking weeks or even months in supercomputer (HPC) architecture. Given the substantial costs involved (both computer infrastructure and time), the natural question arises:
What would be the least/optimal amount of simulations I would need to guarantee an appropriate training dataset for my surrogate model?
As one might expect, the answer eludes even the most experts in the field, given the immeasurable complexity behind real-life mixing system dynamics. Unfortunately, the solution does not come as easy as coupling DoE with simulation deployment, as the runs carried out do not necessarily guarantee sufficient/suitable training data for our surrogate model. The intricacies behind this problem require a higher level of connectivity between different approaches. Quaisr can boost DoE libraries with optimisation algorithms and link them to the simulation and surrogate model pipeline. The DoE generator can receive feedback from the surrogate model at the other end of the pipeline, and optimise its search for the next simulation run accordingly instead of creating a ‘blind’ set of simulation runs. It may seem like years of development are required to have such an interconnected system, but all of this can be achieved today using the Quaisr platform.